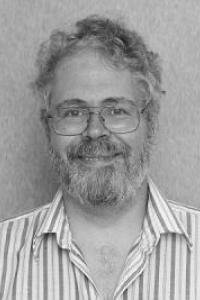
Office: 6721 Gates & Hillman Centers
Email: jc6o@andrew.cmu.edu
Phone: 412-268-7279
Department(s):
Language Technologies Institute
Jaime Carbonell
LTI Director/Professor of CS and LTI
My interests span several areas of Artificial Intelligence and Language Technologies, Machine Learning and Computational Proteomics as detailed below. My research style typically combines theory, experimentation and system building in collaboration with students, research staff, and faculty colleagues. More specifically, the following illustrate my recent research areas:
Proactive Learning: Whereas supervised machine learning methods are effective given sufficient training data, active learning addresses the issue of selecting unlabeled instances, which if labeled would maximize learning effectiveness. Proactive methods generalize active learning into a constrained optimization process, taking into account differential labeling costs, labeling reliability, and selection of labeling oracles: e.g. human expert, lab experiment, large-scale simulation, crowd-sourcing, etc.
Enriched Learning: Machine learning can be improved via transfer learning: prior learning on similar domains or tasks. It can also be improved by enriched teacher-student dialog: going beyond just atomic label queries, typical in active learning. For instance, region queries and similarity queries can reduce sample complexity in certain cases. The goal of this research (with Prof. Avrim Blum) is to go well beyond traditional learning from instance-label pairs.
Machine Translation: I am working on learning-based methods for Machine Translation, especially for rare languages where parallel training text is very scarce. This requires linguistic structures as priors, and learning at the structure level as well as the lexical and phrasal transfer level.
Computational Proteomics: Proteins are the key building blocks of all living organisms, both in terms of structure and function. They are the components or virtually all biological engines, and the target of most drugs. I have studied how to infer 3D spatial structure from 1D sequences via structural machine learning methods. With Prof Judith Klien-Seetharaman, and I now looking at machine learning methods to induce the host-pathogen (e.g. human-HIV or human-salmonella) protein-protein interaction graph, since pathogens infect hosts via complex protein interactions.