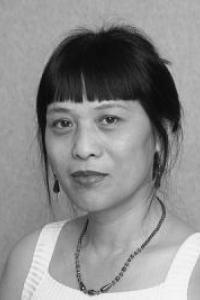
Office: 6717 Gates & Hillman Centers
Email: yiming@cs.cmu.edu
Phone: 412-268-1364
Department(s):
Language Technologies Institute
Yiming Yang
Professor
My research has centered on statistical learning methods/algorithms and application to very-large-scale text categorization, web-mining for concept graph discovery, semi-supervised clustering, multitask learning, novelty-based information retrieval, large-scale optimization for online advertising, social network analysis for personalized email prioritization, etc. My recent research focuses on the following topics:
Large-Scale Structured Learning for Hierarchical Classification (Gopal & Yang, KDD 2013; Gopal & Yang, ICML 2013 & Supplementary ; Gopal et al., NIPS 2012)
- Providing organizational views of multi-source Big Data (e.g., Wikipedia, online shops, Coursera)
- State-of-the-art classifiers for large-scale classification over hundreds of thousands of categories
- Scalable variational inference for joint optimization of one trillion (4 TB) model parameters
Scalable Machine Learning for Time Series Analysis (Topic Detection and Tracking)
- From scientific literature, news stories, sensor signals, maintenance reports, etc.
- Modeling multi-source and multi-scale evidence of dynamic chances in temporal sequences. (On-going NSF project; Gopal, PhD Thesis)
- A new family of Bayesian von Mices Fischer (vMF) clustering techniques (Gopal & Yang, ICML 2014 & Supplementary)
- Unsupervised clustering and semi-supervised metric learning and supervised classification (Gopal & Yang, UAI 2014 & Supplimentary).
Concept Graph Learning for Online Education (NSF project; Yang et al., WSDM 2015)
- Mapping online course materials to Wikipedia categories as the Interlingua (universal concepts)
- Predicting conceptual dependencies among courses based on partially observed prerequisites
- Planning customized curriculum for individuals based on backgrounds and goals
Macro-Level Information Fusion for Events and Entities (joint effort with Jaime Carbonell in the DARPA DEFT project)
- Detecting entities and events of interest in various forms of mentions in text to enable high-precision, semi-structured information fusion and summarization. Using a corporate acquisition event as an example, different (and partially redundant) sentences can mention acquirer, price, date, approvals, joint-management, etc. This multi-aspect information needs to be jointly extracted into a unified structured form for this event type, with uncertainty estimates in the aggregated representation.